Study AI drug discovery Start with these 3 papers
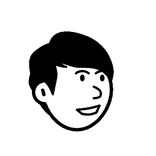
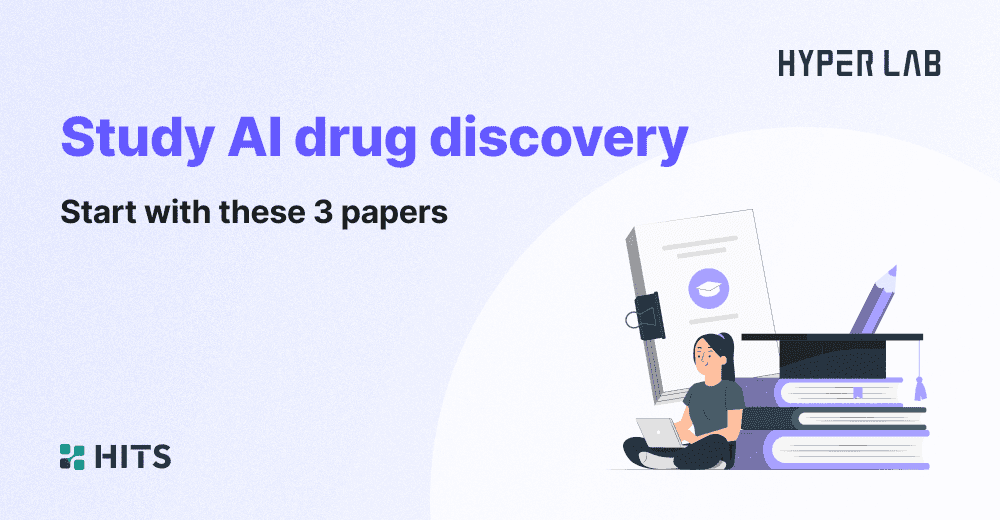
AI drug discovery is a rapidly evolving field. To help students and researchers who are struggling to find a starting point amidst the daily flood of AI papers, we've curated three important papers that provide key insights and foundations.
While these papers are not cutting-edge, we believe they provide an excellent starting point for understanding the basics of AI drug discovery research and moving on to more cutting-edge work.
We hope they give you a clear understanding of how rapidly the field is evolving and provide a foundation for deeper learning.
Papers to get you started in AI drug discovery
Paper Summary : Using generative AI to kick-start AI drug discovery research
Paper Resources : Automatic Chemical Design Using a Data-Driven Continuous Representation of Molecules
This innovative paper, published in 2018, breaks new ground in molecule generation by utilizing a Variational Autoencoder (VAE). The researchers were the first in the world to demonstrate that deep learning models can generate chemically valid molecules.
Molecules are made up of atoms, which are connected by chemical bonds. However, only a small fraction of the myriad arrangements of atoms are chemically meaningful, and this research demonstrates that deep learning models can learn the rules of these arrangements.
Furthermore, by using a Gaussian Process to regulate the latent space, the researchers succeeded in generating molecules with the desired properties, which opens up the possibility of generating molecules that satisfy specific pharmacological properties in drug discovery. The figure below is an example of molecules generated using this method.
Since the publication of this paper, various studies using generative AI have been published, paving the way for molecular generation models. It is highly recommended for those who are curious about the starting point of generative AI in the field of AI drug discovery.
Papers that made AI drug discovery a real possibility
Paper Summary : Generating preclinical candidates in 46 days using AI.
Paper Resources : Deep learning enables rapid identification of potent DDR1 kinase inhibitors
As with any field of scientific research, it is very difficult to go beyond benchmark tests and demonstrate the performance of AI drug discovery models in real-world experiments. In this context, a paper that uses AI to identify preclinical candidates in just 46 days is particularly noteworthy. This paper is a great example of how AI can be applied to real-world drug discovery.
The authors of the paper utilized reinforcement learning techniques to design DDR1 kinase inhibitors. They synthesized the designed molecules, validated their activity through biochemical and cell-based assays, and conducted pharmacokinetic testing in rats. The figure below is a timeline of these 46 days.
It should be noted, however, that the generative AI used in the study did not account for all the properties tested, and that the molecules generated were structurally very similar to previously reported drugs. It is also important to note that not all drug discovery tasks can be accomplished this quickly with AI.
Papers on AI drug discovery and other challenges in science
Paper Summary : AI enables three-dimensional structure prediction of protein sequences.
Paper Resources : Highly accurate protein structure prediction with AlphaFold
Predicting three-dimensional structures from protein sequences has long been a challenge for the scientific community. A monumental breakthrough was made by DeepMind's AI, AlphaFold, which is self-explanatory and has revolutionized the field of protein structural biology.
The structure of the AlphaFold model is simply summarized as follows. First, the coevolutionary information of the amino acid sequence is extracted through Multiple Sequence Alignment (MSA). Then, we use a deep learning model based on Transformer to extract interaction information between amino acid pairs. In the final step, we predict the structural arrangements (distances and angles) between residues and reconstruct the 3D structure of the protein from the predicted information.
To fully understand this model, you need a deep knowledge of deep learning and an understanding of basic structural biology. If you can fully grasp this paper, you are a master in the field of deep learning + structural biology.
Wrapping up
In this article, we've covered some of the best resources for studying AI drug discovery. Aren't you excited about the future of AI-accelerated drug discovery? Now that you've learned about the advancements in AI technology through academic papers, let's take a look at Alphafold. If you're interested in learning more about Google DeepMind's Alphafold, check out these posts