Google DeepMind AlphaFold-latest l What's different from previous AlphaFolds, how they differ
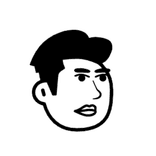
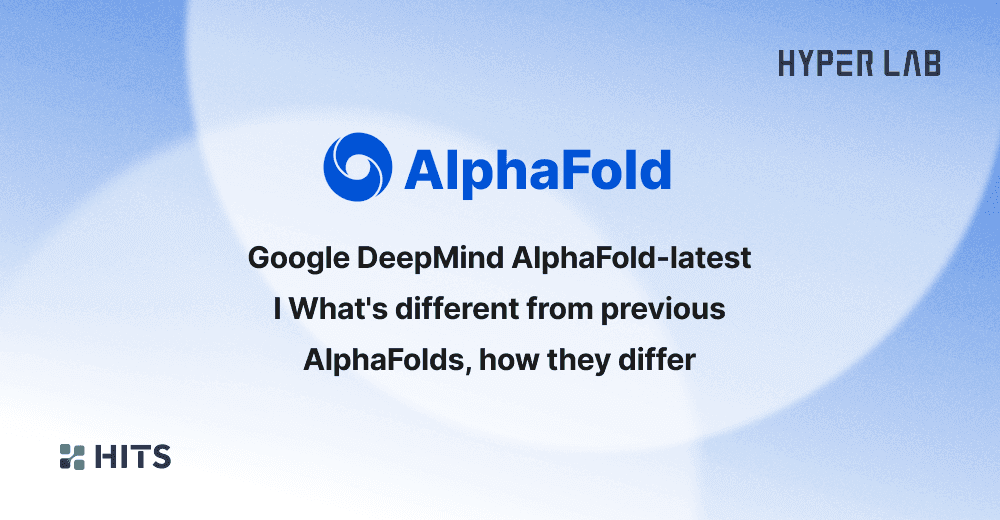
Even if you're not familiar with artificial intelligence technology, you're probably not familiar with AlphaFold
AlphaFold, which predicts three-dimensional structures from protein sequences, has made such an impact on the scientific community that its main developers, Demis Hassabis and John Jumper, were awarded Breakthrough Prize and the Lasker Award in 2023. Since the opening of the AlphaFold Database, it has become commonplace to use the "AlphaFold predicted structure of a target protein" in drug discovery projects.
AlphaFold progressively predicts the structure of a multi-domain protein (CASP14 T1091) [Nature 596: 583 (2021), CC BY 4.0
AlphaFold has steadily evolved from Version 1 (2018), to Version 2 (2020), to AlphaFold-Multimer (2021), to the most recent Version 2.3 (2022), increasing its predictive protein size and accuracy.
On August 31, 2023, Google DeepMind shared news of the next generation of AlphaFold. Dubbed AlphaFold-latest, this model is setting off alarm bells for researchers in related fields, just as it did when AlphaFold's performance was first revealed. But what's different?
Google DeepMind AlphaFold-latest What's different?
When comparing Google DeepMind's AlphaFold-latest to the last publicly available model, AlphaFold 2.3, the biggest change is that it can now predict almost all biomolecular structures, including proteins, small molecules, DNA and RNA, ions, and modified amino acids.
Biomolecule structures predicted by AlphaFold-latest. In each example, AlphaFold-latest's predictions are colored in cyan and the experimentally observed structures are colored in white.
Differences between AlphaFold and Alphafold-latest
Whereas AlphaFold could only predict protein structures, AlphaFold-latest can now predict almost any molecular combination needed for drug discovery, including not only isolated nucleic acids, but also protein-nucleic acids, protein-small molecule compounds, and protein-antibodies. This is an incredible advancement because it allows us to better understand how drugs work than ever before.
Google DeepMind AlphaFold-latest surprises.
1. AlphaFold-latest has high prediction accuracy
Predicting the structure of a protein-ligand complex has been an intractable problem for a very long time. Any drug discovery researcher will agree with the need for this research.
While a variety of deep learning models for predicting protein-ligand complex structures have recently been published in academia, the standard industry tool is still a docking program. These are programs that use highly approximated physicochemical laws and classical algorithms to explore non-protein (mostly small molecules) binding structures.
AlphaFold-latest is more than 40% more accurate than the best docking programs for predicting the structure of protein-ligand complexes alone, with an accuracy of 37-53% for covalently bound non-proteins such as covalent ligands, glycoproteins, modified amino acids, and modified nucleic acids, which are particularly challenging to predict. Complex protein structures, such as protein multimers and protein-antibody conjugates, have also been reported to show significant accuracy improvements over the previous AlphaFold 2.3.
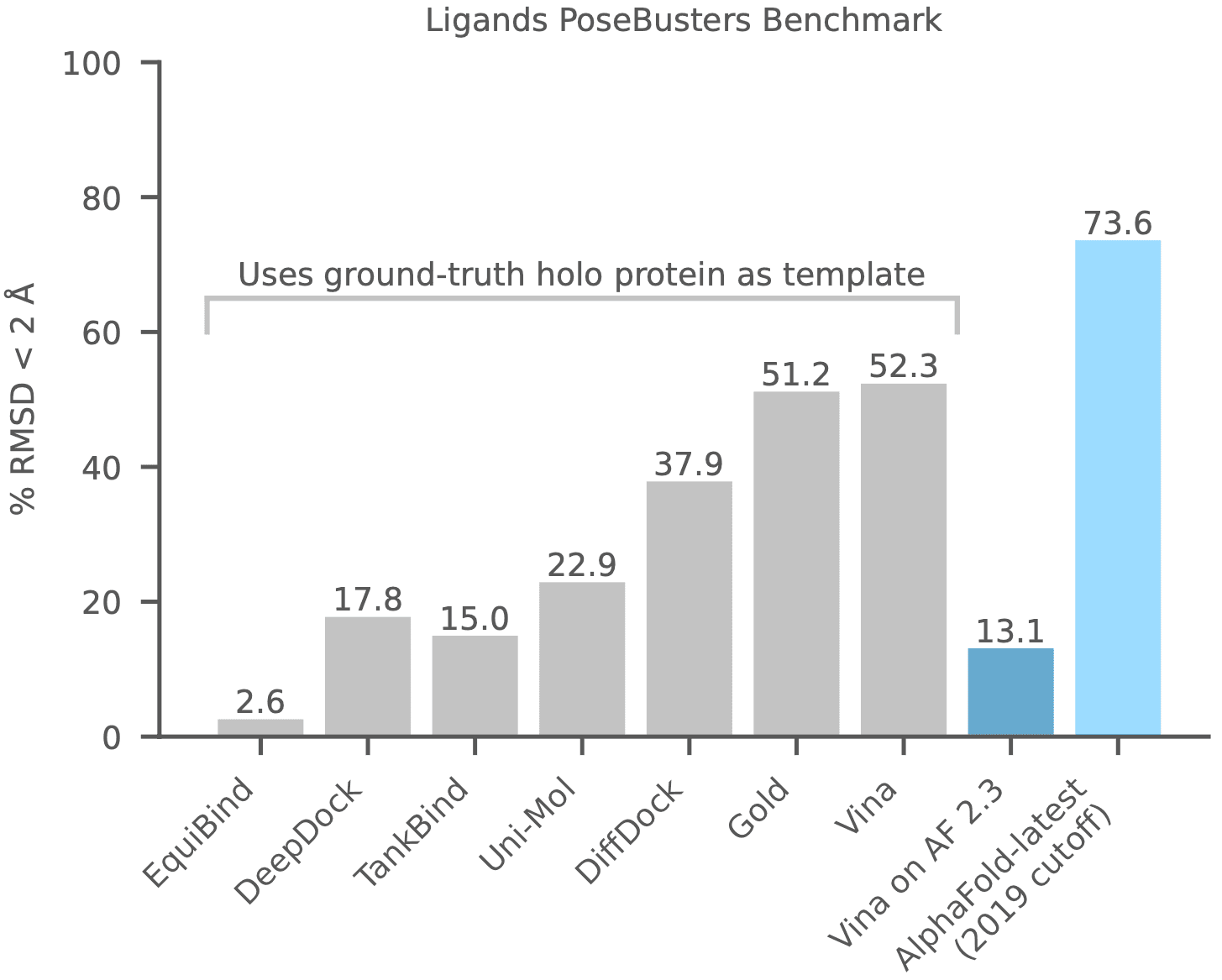
[PoseBusters benchmark].
Deep learning models including EquiBind, DeepDock, TankBind, Uni-Mol, DiffDock, etc.
docking programs such as Gold and Vina are compared to AlphaFold-latest.
The AlphaFold-latest model was trained on data prior to 2019-09-30.
[Google DeepMind & Isomorphic Labs, 2023].
The ability of AlphaFold-latest to predict a variety of new protein-non-protein complex structures can be seen in the figure below.
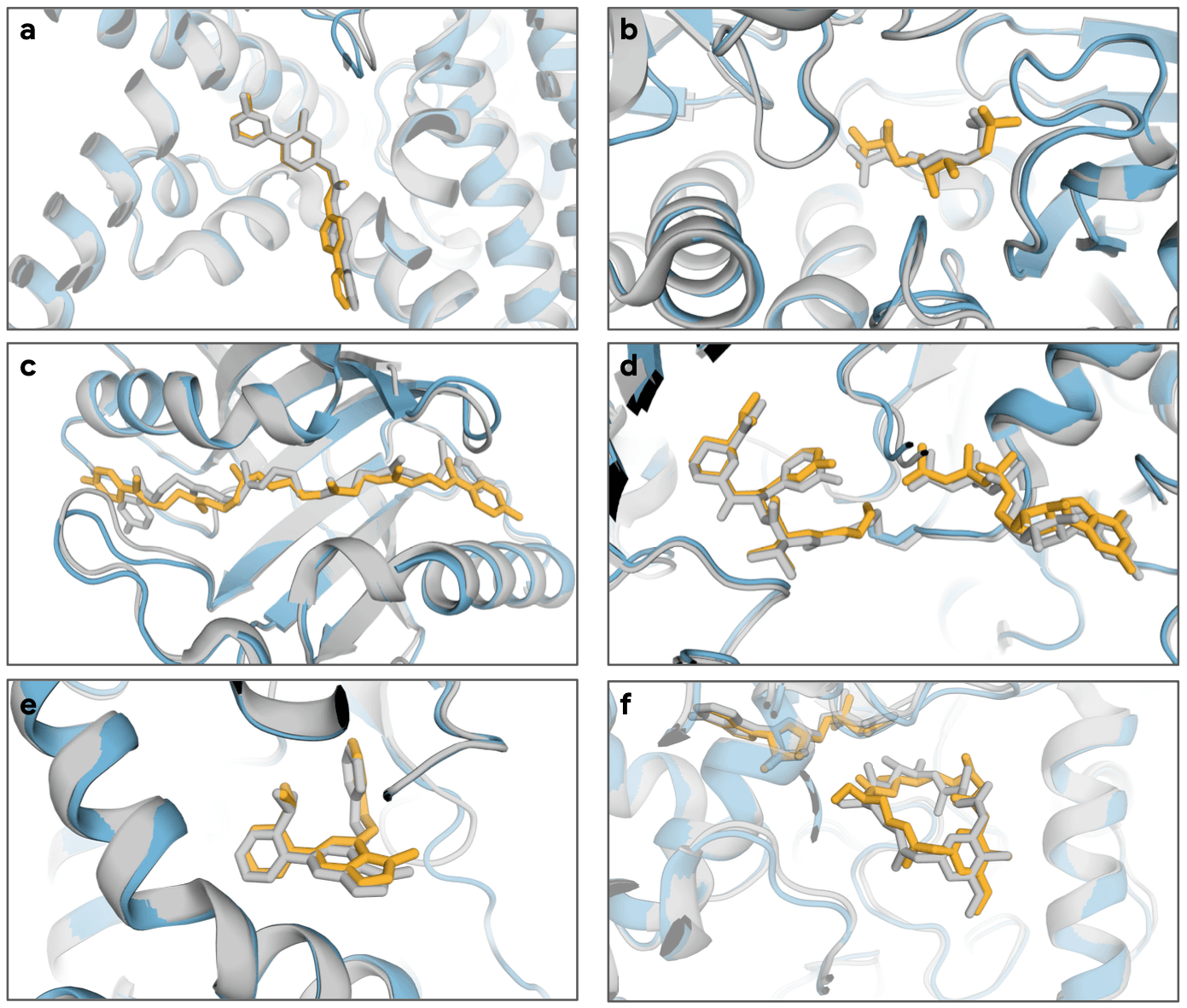
(a) LGK974 bound to the PORCN-WNT3A complex (PDB ID 7URD).
(b) (5S,6S)-O7-sulfo DADH bound to AziU3/U2 (PDB ID 7WUX).
(c) Closthioamide bound to CtaZ (PDB ID 7ZHD).
(d) Sanglifehrin A analog covalently bound to KRAS G12C and CypA (PDB ID 8G9Q).
(e) NIH-12848 analog that binds to the allosteric site of PI5P4Kγ (PDB ID 7QIE).
(f) 20-O-methyl-19-chloroproansamitocin macrocycle ligand and cofactor bound to GdmN (PDB ID 7VZN)
[Google DeepMind & Isomorphic Labs, 2023].
2. AlphaFold-latest with maximum output relative to minimum input
It's great to be able to make accurate predictions across a wide range of categories, but does it require a lot of input?
The most amazing thing about Google DeepMind AlphaFold-latest is that the information it requires is simple: just a protein/nucleic acid sequence and a ligand text representation (SMILES string). It doesn't even need a three-dimensional protein structure as a reference, or even binding location information. In contrast, the docking programs compared above need to know the three-dimensional structure of the target protein, as well as the location and extent of binding.
Predicting the three-dimensional structure of a complex from textual information alone means predicting protein conformational changes due to ligand binding. This means that while most docking programs and deep learning models ignore protein conformational changes, AlphaFold-latest takes into account the fluidity of the binding site to match the ligand it binds. This is important not only when the ligand is a small molecule, but also when it is another protein or antibody.
Google DeepMind AlphaFold-latest Substitutability.
Similar deep learning model RoseTTAFold
A model often discussed alongside AlphaFold is [RoseTTAFold] (https://www.science.org/doi/10.1126/science.abj8754) from David Baker's group at the University of Washington, USA. RoseTTAFold is a similar deep learning model that predicts three-dimensional structures from protein sequences.
The two models were released around the same time. AlphaFold 2 was published in Nature in July 2021, and RoseTTAFold was published in Science in August of the same year. Then, in November 2023, Baker's group introduced [RoseTTAFold2NA] (https://doi.org/10.1038/s41592-023-02086-5), which predicts the binding structure of protein-nucleic acid complexes.
We mentioned above that AlphaFold-latest also predicts protein-nucleic acid complex structures. The Google DeepMind announcement also included a performance comparison between AlphaFold-latest and RoseTTAFold2NA. The results show a clear victory for AlphaFold-latest, as shown in the figure below.
AlphaFold-latest VS RoseTTAFold2NA
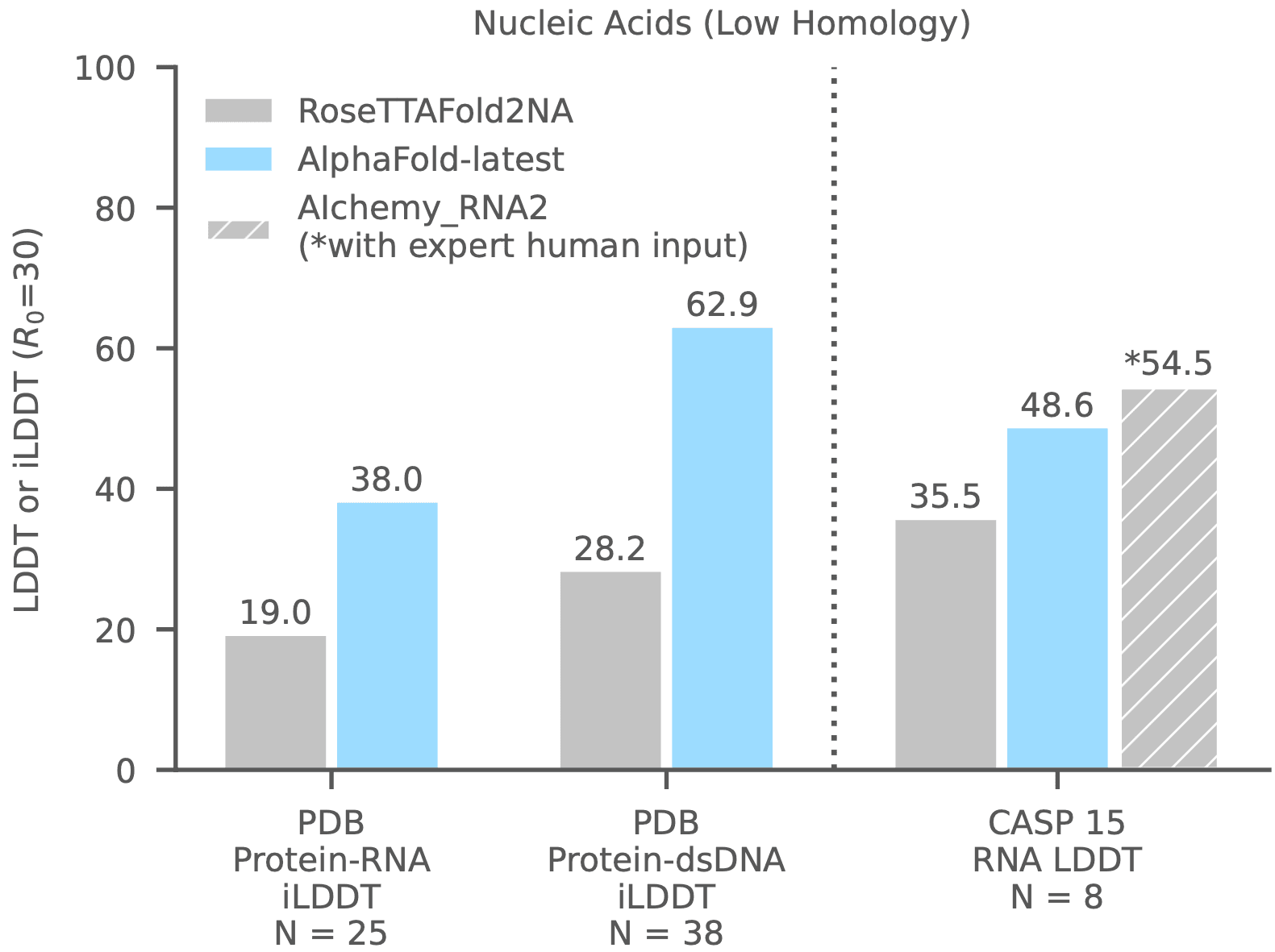
AlphaFold-latest VS RoseTTAFold All-Atom
Coincidentally, on August 9, 2023, a little before AlphaFold-latest was reported, a preprint of RoseTTAFold's next generation model, RoseTTAFold All-Atom, was published. It is very similar in functionality to AlphaFold-latest, including 1) accepting only textual information such as sequences and SMILES as input, and 2) including non-proteins such as nucleic acids, ions, and small molecules.
Furthermore, compared to AlphaFold-latest, which only lists results, the full manuscripts that are presumably under review by journals are made public. The structure and training of the model is also explained in detail. To summarize the performance, AlphaFold-latest is worse than docking programs such as Vina, Gold, etc. in the same benchmark (*40% structure prediction success rate) **This means that AlphaFold-latest is better than RoseTTAFold All-Atom for predicting ligand-binding structures.
Google DeepMind also reported as follows.
During preparation of this manuscript, independent work on RoseTTAFold All-Atom (Krishna et al., 2023) was released that performs structure prediction and protein design across a wide range of biomolecular systems. This system is not available for baselining at the time of writing, but the RoseTTAFold All-Atom paper indicates their accuracy is below specialist predictors in almost all categories.
An important aspect of the RoseTTAFold preprint is that it also introduces an All-Atom version of RFdiffusion, a protein generation model. RFdiffusion was previously published in the July 2023 issue of Nature (https://doi.org/10.1038/s41586-023-06415-8), and like RoseTTAFold All-Atom, RFdiffusion All-Atom has been extended to generate proteins that can bind to non-proteins, such as small molecules. We're excited to see what amazing applications RFdiffusion All-Atom will bring to the table in the future, independent of AlphaFold-latest or RoseTTAFold All-Atom.!
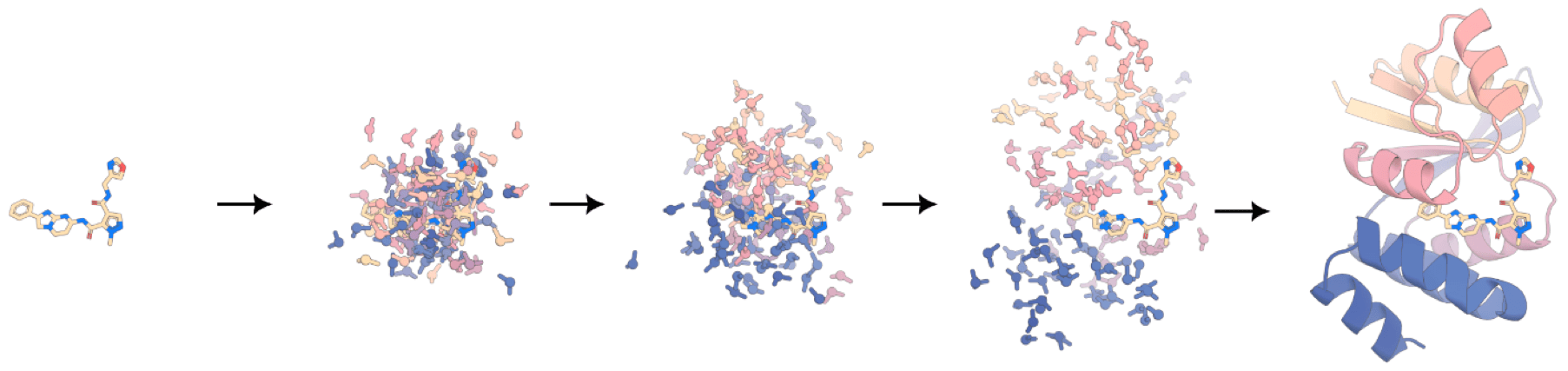
Why Google DeepMind's move is important to note
After reading the latest AlphaFold results from Google DeepMind, you might be thinking, "The development of AI technology to target small molecule drugs is over." Is that really the case?
AlphaFold-latest announcement leads to better technology development
As we've seen, AlphaFold-latest is clearly the next big thing after AlphaFold. For example, the recent Isomorphic Labs' $4 trillion research collaboration deal with Eli Lilly and Novartis is a testament to the power of the Alphafold model.
But there's still a lot of work to be done. First, it's important to recognize that many of the performance metrics in this report fall far short of a perfect score of 100. Even if the problem of predicting protein-ligand binding structures is solved, there are still many places in small molecule drug discovery where computational techniques are needed. Activity prediction at various stages: enzymatic, cellular, tissue, disease model, clinical, etc. And then there's pharmacokinetics, which has a long way to go.
And AlphaFold-latest won't just impact small molecule targeting: as we've seen, AlphaFold-latest improves on AlphaFold 2.3 in terms of protein structure prediction, and will have a significant impact on problems where protein-protein interactions are important, such as antibody design.
It's unclear if Google will ever fully release AlphaFold-latest, and if so, when. But at the very least, we can expect that if models like RoseTTAFold All-Atom are made available to academia, the state of the art and accessibility of biomolecular structure prediction will become much better.
Just as open sources including Llama 2 were developed in response to GPT and competed with each other to improve the performance of deep learning models, it will be interesting to see what Google DeepMind does in the future with our Hyperlab.