Innovation and Future of Ultra-large Compound Libraries
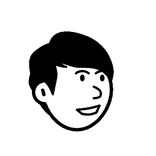
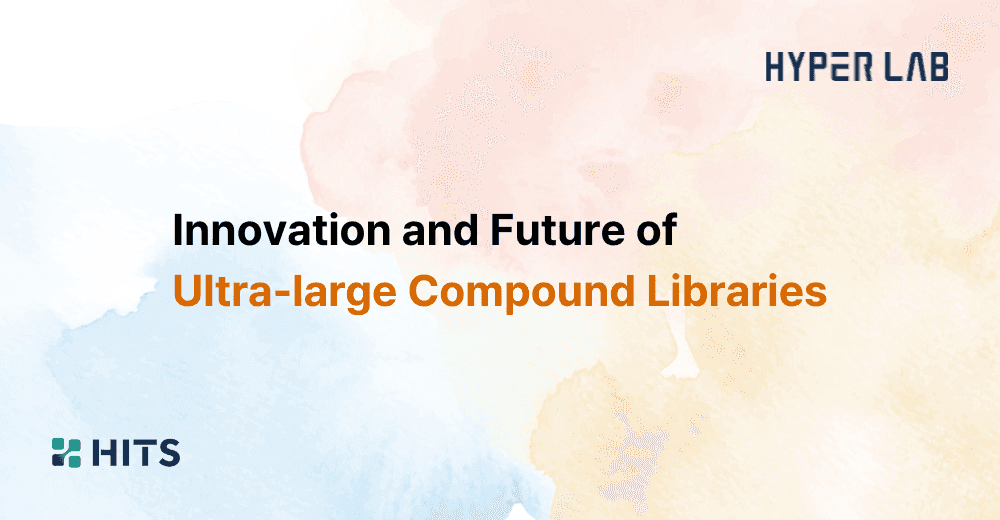
Emergence and Importance of Ultra-large Compound Libraries
Ultra-large libraries (referred to hereafter as virtual libraries) are massive compound databases used in drug discovery, typically containing hundreds of millions to trillions of compounds. In contrast to traditional compound libraries, which usually consist of thousands to tens of thousands of compounds, these libraries represent a revolutionary advancement. Enabled by breakthroughs in DNA-encoded library (DEL) technology and AI-driven virtual screening techniques, virtual libraries encompass an extensive range of chemical structures, from synthesizable compounds to entirely virtual ones. Their scale provides unprecedented chemical diversity and possibilities for drug developers. When integrated with artificial intelligence, virtual libraries greatly enhance the efficiency of identifying novel drug candidates.
Principles and Applications of Virtual Libraries
Virtual libraries are constructed by combining building blocks and reaction templates. Building blocks are inexpensive, stable, and reactive small molecules, while reaction templates are straightforward, high-yield chemical reactions. For instance, with 10,000 building blocks and 10 reaction sets, one can theoretically generate up to 10,000 × 10,000 × 10 = 10¹¹ virtual compounds. Although some reactions may not work with certain building block combinations, the number remains astronomically high.
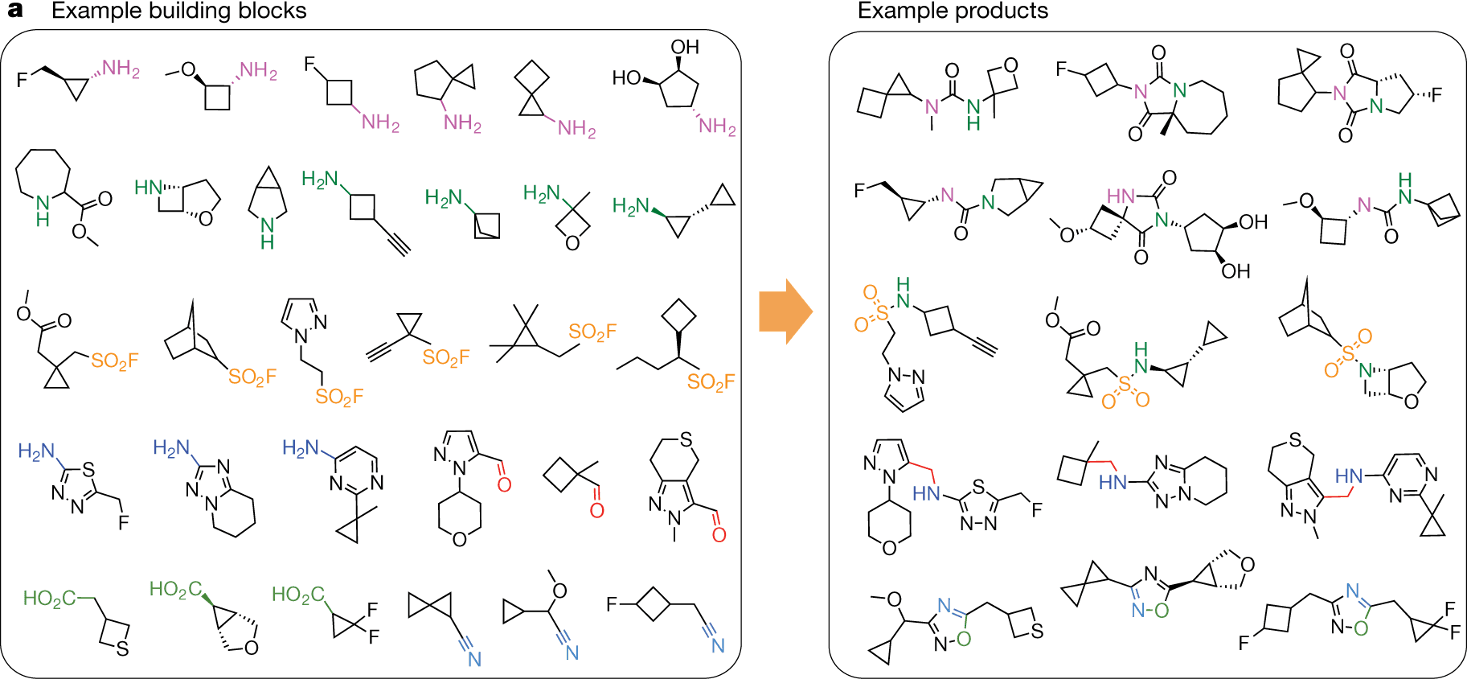
This vast size overcomes the limitations of traditional high-throughput screening (HTS), which typically evaluates libraries of about a million compounds, dramatically increasing the chances of successful drug discovery. A key advantage of virtual libraries is their ability to improve hit rates. Even with the same screening methods, larger libraries yield higher hit rates. Intuitively, it’s like comparing the probability of finding the top student in a school (with hundreds of students) versus in a single class (with only dozens).
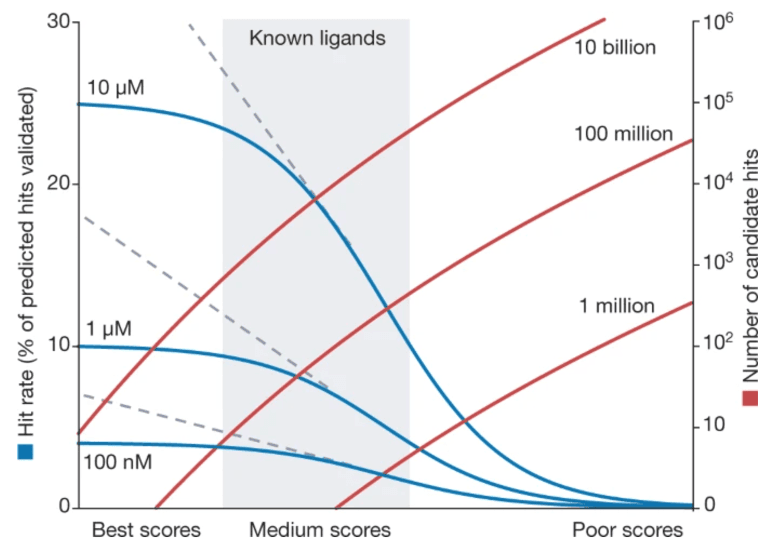
Innovation and Applications of DNA-encoded Libraries (DEL)
The advent of DNA-encoded library (DEL) technology has been critical to building ultra-large libraries. DEL involves attaching unique DNA barcodes to compounds, enabling the simultaneous synthesis and screening of billions of compounds. Companies like X-Chem have built DEL libraries containing over 200 billion compounds, with ongoing expansions. Research shows that DEL-identified hits often exhibit unique binding modes, uncovering opportunities unavailable through traditional methods (Reference: ACS Journal of Medicinal Chemistry).
Recent research demonstrates that ultra-large libraries not only boast an enormous number of compounds but also enable efficient exploration of broader chemical space. Combining Diversity-Oriented Synthesis (DOS) techniques with DEL has made it possible to synthesize compounds with unique characteristics that are absent from commercial screening libraries. For instance, one research group built a DEL comprising 3.7 million structurally diverse compounds and made it publicly available to accelerate early drug discovery (Reference: https://pubmed.ncbi.nlm.nih.gov/37582753).
Advancements in Machine Learning and AI-based Screening
Machine learning (ML) has further enhanced the utility of ultra-large libraries. Recent studies have successfully combined ligand-based (LB) and structure-based (SB) approaches to predict protein-ligand interactions more accurately. AI-driven technologies can now screen billions of compounds within minutes, significantly reducing false positives (Reference).
For instance, tools like HASTEN integrate ML predictions with docking simulations to improve screening throughput. A case study showed that screening just 1% of a library using docking simulations identified 90% of the top 1,000 virtual hits (Reference).
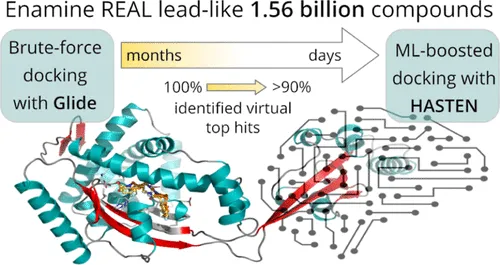
Ultra-large libraries have shown remarkable success in identifying effective compounds for ion channel targeting, leading to breakthroughs in treatments for diseases like diabetes, epilepsy, hypertension, cancer, and chronic pain. Advances in computational hardware and software have further amplified the efficacy of these screening efforts (Reference).
Notably, ultra-large libraries played a pivotal role in developing inhibitors for the SARS-CoV-2 main protease (Mpro). AI-driven screening identified effective inhibitors from billions of compounds, highlighting the synergistic power of AI and ultra-large libraries (Reference).
Challenges and Future Prospects of Ultra-large Libraries
Despite their rapid evolution and powerful capabilities, ultra-large libraries face several challenges. Developing methodologies for more challenging targets, selecting and utilizing chemical space effectively, and addressing scalability issues all remain areas for improvement. Overcoming these challenges will position ultra-large libraries as a transformative tool in increasing the success rate of drug discovery.
These developments and challenges represent a new paradigm in drug discovery, with ongoing innovation promising even greater advances. As AI technologies continue to evolve, the efficiency and accuracy of ultra-large libraries are expected to improve, solidifying their role as a cornerstone of modern drug discovery.