The First AI-Physics Hybrid Docking: Advancing Drug Development Accuracy
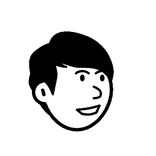
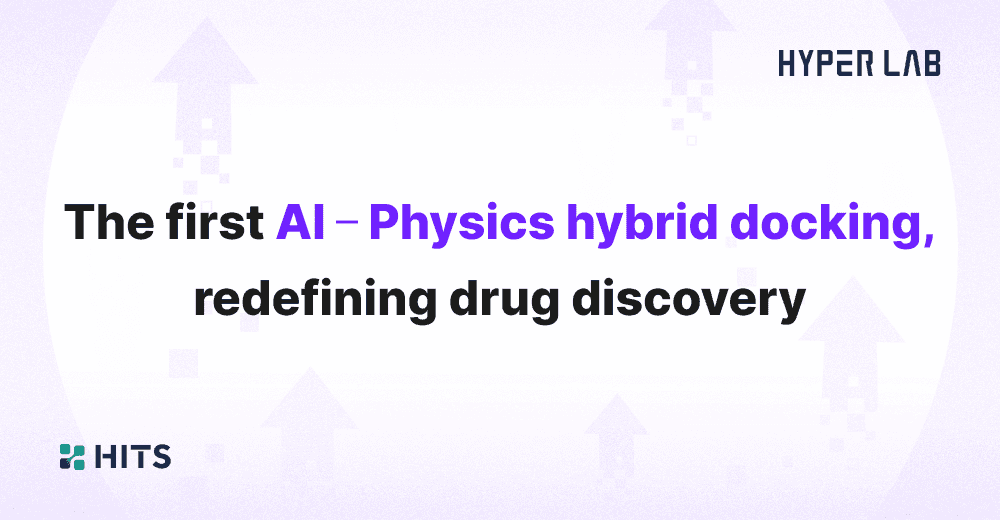
Predicting ligand-protein binding affinity is one of the most crucial technologies for effective drug discovery, particularly in identifying hit compounds during the early stages of drug development. For the past 30 years before deep learning technologies emerged, molecular docking has been the most widely utilized approach for this purpose. You may be familiar with prominent docking software such as Schrödinger's Glide, Discovery Studio's GOLD, and AutoDock Vina.
Despite the availability of these useful docking tools, many experimental researchers regarded docking merely as a "supplementary tool" due to its low accuracy. The emergence of deep learning-based docking methods (or AI docking for short) has garnered attention as a promising alternative that could change this perception, demonstrating significant improvements in accuracy.
However, despite the rapid advancement of deep learning technology, a fundamental limitation of existing AI docking methods is their notably poor performance in regions where training data is scarce. The key to solving this challenge can be found in conventional docking methods. The solution lies in combining the strengths of both deep learning and traditional docking approaches while complementing their respective weaknesses.
This innovative idea led to the development of PIGNet, which stands as the only method that simultaneously achieves both high generalization capability and accuracy in predicting ligand-protein interactions. Let's delve into how PIGNet managed to achieve such remarkable performance.
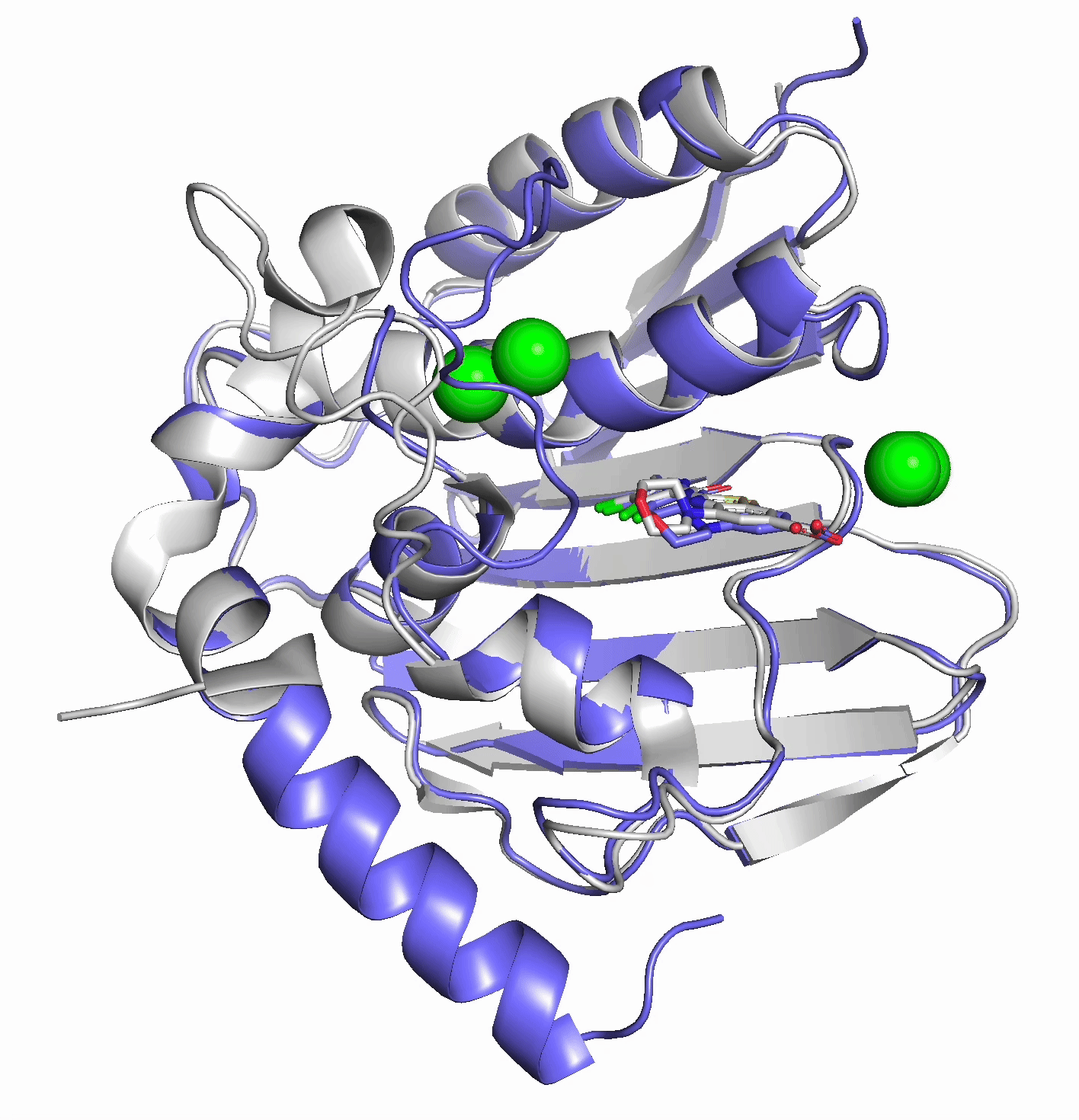
Comparison of Physics-Based and Data-Driven Approaches
Molecular docking and molecular dynamics simulations predict ligand-protein interactions based on physical laws. These methods consider various atomic-level interactions that researchers can interpret, such as hydrogen bonds, hydrophobic interactions, and van der Waals interactions. Since physical laws are universal, these methods are likely to maintain their accuracy for novel molecular and protein structures. Moreover, they allow us to interpret why certain molecules are predicted to have strong binding energies.
However, the drawback of physics-based methods lies in their computational demands: accurate physical simulations lead to exponential increases in computation time, while using approximations to reduce computational cost significantly compromises accuracy. As a result, methods like FEP+, while relatively accurate, require substantial resources and time. In particular, this limitation poses a significant constraint in virtual screening. Additionally, these methods often require complex initial setup and extensive prior knowledge. When using approximation methods like docking to address these issues, calculation speed improves dramatically, but accuracy decreases considerably.
Data-driven approaches, such as deep learning, learn correlations directly from data. Without explicit physical laws, they extract necessary features from ligand-protein binding structures and learn their relationships with binding energies. Since these relationships are automatically learned from data, they don't require physical approximations and, consequently, avoid approximation errors. They also offer impressive speed advantages.
The most significant limitation of data-driven approaches is their potential performance degradation when sufficient data isn't available. While they show high performance for molecules similar to their training data, their performance drops dramatically for dissimilar molecules. This becomes a critical weakness in drug discovery, where the goal is to identify novel compounds that haven't been previously reported.
As we can see, both approaches have their distinct advantages and disadvantages. This raises an important question: Could we combine these two approaches to complement each other's strengths and weaknesses? This was the motivation behind developing PIGNet (Physics-Informed Graph Neural Network).
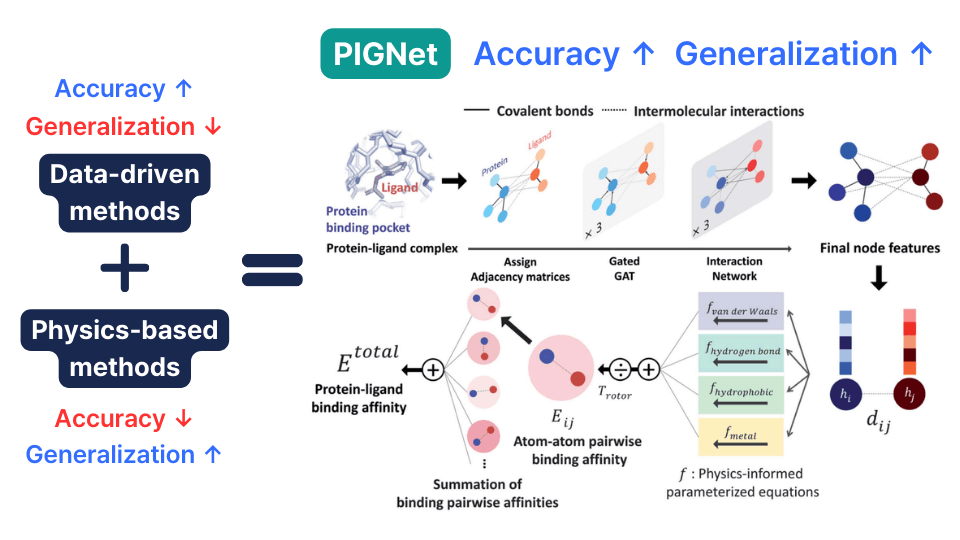
PIGNet: Combining the Advantages of Physics-Based and Data-Driven Approaches
PIGNet (Physics-Informed Graph Neural Network) overcomes the limitations of physics-based approximations by adopting deep learning methodology while mitigating the overfitting problems of data-driven approaches through physical laws. Instead of directly predicting the output (binding affinity), PIGNet predicts the parameters that go into physical equations. The final prediction is calculated using these predicted parameters from the deep learning model in conjunction with physical equations. First, the van der Waals energy, one of the non-bonding interactions, is calculated using an equation. This equation uses the Lennard-Jones potential to calculate interaction energy based on inter-atomic distances.
\[ E^{\text{vdW}} = \sum_{ij} c_{ij} \Bigg[\Bigl(\frac{d_{ij}'}{d_{ij}}\Bigr)^{12} - 2 \Bigl(\frac{d_{ij}'}{d_{ij}}\Bigr)^6\Bigg] \]
Hydrogen bonds, hydrophobic interactions, and metal-ligand interactions are calculated using another equation.
\[ e_{ij} =
\begin{cases}
w, & \text{if } d_{ij} - d_{ij}' < c_1,\\
w \Bigl(\frac{d_{ij} - d_{ij}' - c_2}{c_1 - c_2}\Bigr), & \text{if } c_1 < d_{ij} - d_{ij}' < c_2,\\
0, & \text{if } d_{ij} - d_{ij}' > c_2,
\end{cases} \]
Finally, the total energy is calculated by summing all interaction energies and dividing by a normalization factor that considers the rotational degrees of freedom of the molecule.
\[ E^{\text{total}} = \frac{E^{\text{vdw}} + E^{\text{hbond}} + E^{\text{metal}} + E^{\text{hydrophobic}}}{T_{\text{rotor}}}. \]
The deep learning model is trained to optimize the accuracy of the final prediction, and after training, these parameters are predicted by the model during inference. In the next section, we will examine PIGNet's performance from two perspectives: predicting binding affinity of derivatives and virtual screening
Performance 1: Predicting binding affinity of derivatives
The prediction accuracy for derivative activities measures how precisely a method can predict the activities of various derivatives. This accuracy is quantified through the Pearson correlation between experimental values (expressed as IC50, Ki, etc.) and predicted values. The closer this correlation value is to 1, the more accurate the prediction. As shown in the graph, PIGNet demonstrates more than twice the accuracy compared to other leading docking methodologies.
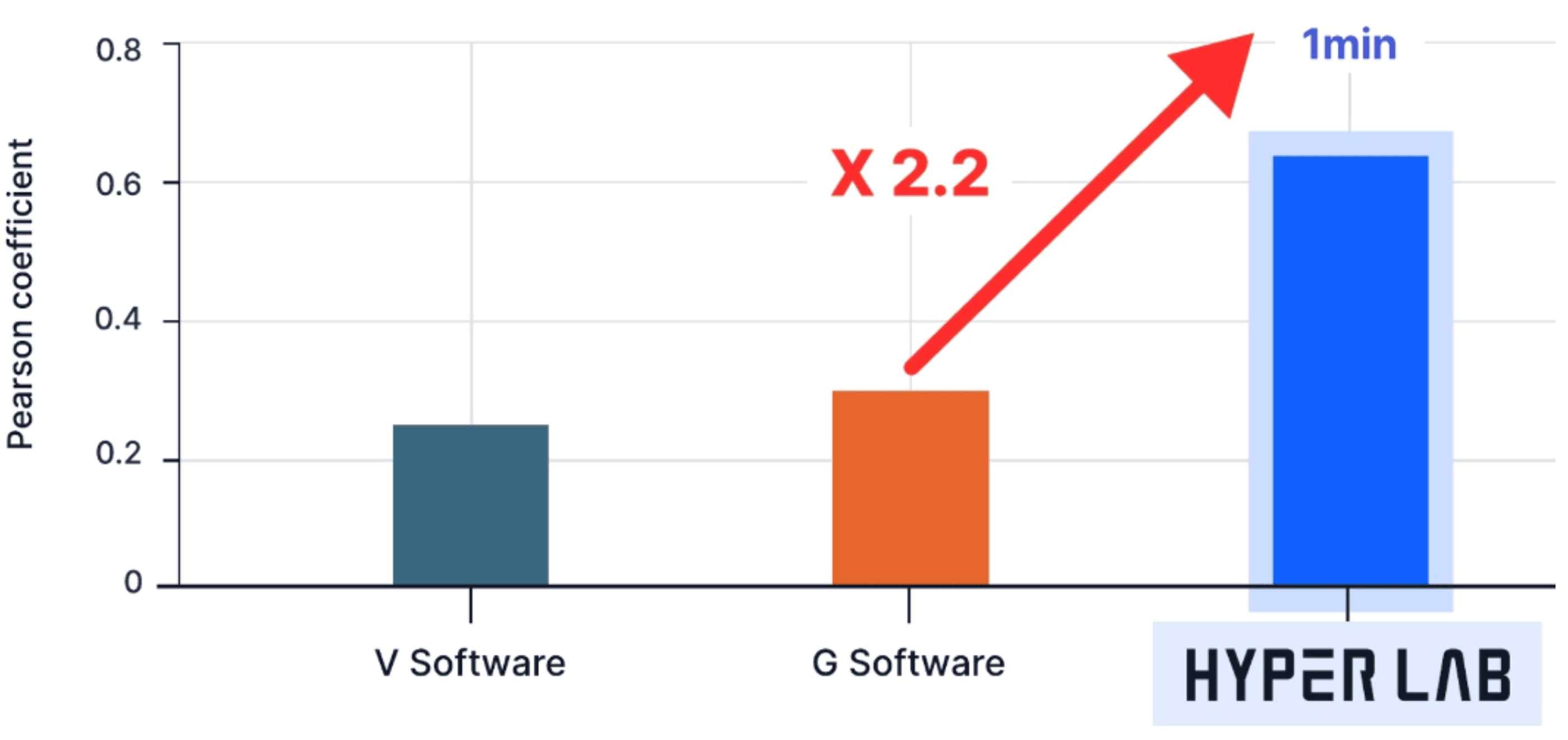
reference: Digital Discovery, 2024, 3, 287-299
Benchmark set: J. Chem. Inf. Model., 2020, 60, 5457–5474
Performance 2: Virtual Screening
The performance of virtual screening can be measured through the enrichment factor (EF). As illustrated in the graph below, PIGNet shows up to twice the EF across various datasets compared to conventional docking methods. A twofold increase in EF indicates that the probability of identifying active compounds in virtual screening is twice as high.
Try PIGNet for free at Hyper Lab
We have explored the theoratical background and performance results of PIGNet, a model that combines rapidly advancing deep learning technology with physical knowledge accumulated over the past century. By addressing the limited data challenge in drug discovery, PIGNet demonstrates the highest accuracy achieved to date. PIGNet serves as the core module of the Hyper Binding feature at Hyper Lab. Experience the most accurate ligand-protein interaction prediction model at Hyper Lab.
Start your Free-trial: https://buly.kr/7mBBcJI
Schedule an Meeting: https://abit.ly/6tr1uz