See the future of AI drug discovery at NeurIPS 2023
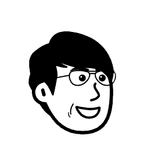
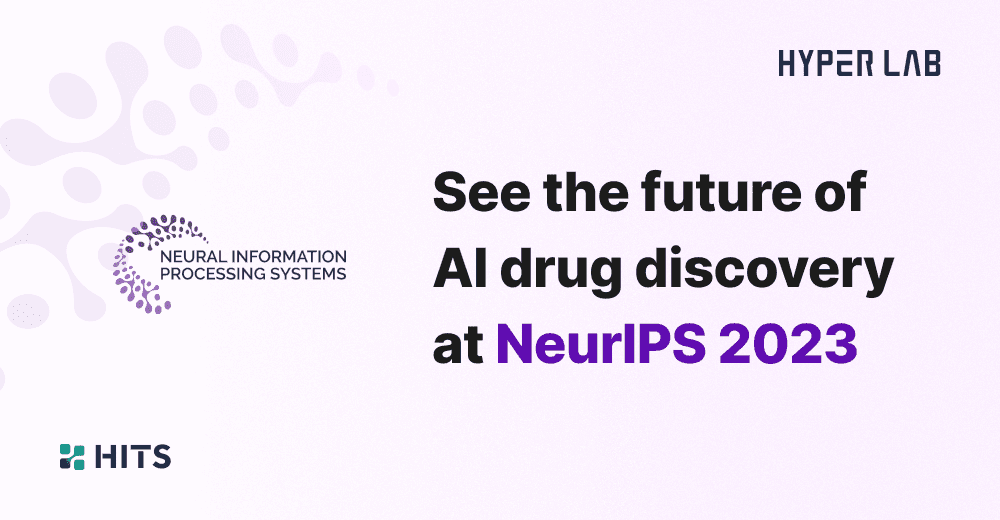
Now in its 37th year, NeurIPS is the world's largest artificial intelligence conference and the best place to see the latest research trends in the field. NeurIPS 2023 covered a lot of topics related to drug discovery, and from this perspective, we will share our impressions of the conference and imagine the future of drug discovery that AI will change.
What is NeurIPS 2023?
NeurIPS stands for Conference and Workshop on Neural Information Processing Systems and was previously known simply as NIPS. As the title suggests, the conference is dedicated to understanding neural network systems, and was founded in 1986 in the United States. In the early days, the society covered both biological and artificial neural networks, but nowadays, it mainly focuses on AI research based on artificial neural networks, which is the basis of deep learning.
It is interesting to note that AI for Science has emerged as an important research topic with the recent advancement of deep learning, and biological research, including drug discovery, is rapidly gaining momentum. In this article, I would like to introduce my experience of traveling with KAIST students to present two papers at the NeurIPS 2023 conference held in New Orleans, USA in December.
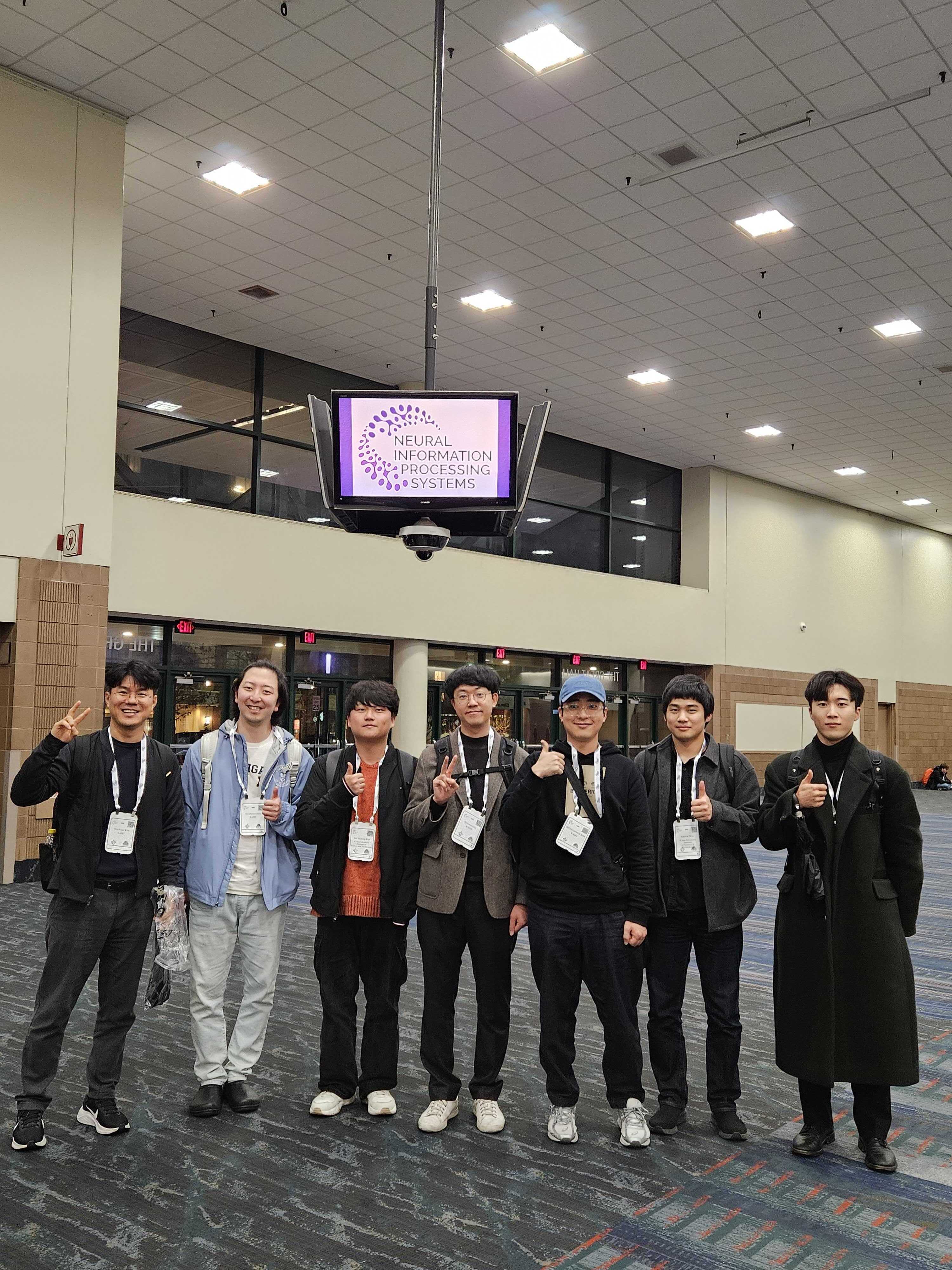
The future of drug discovery as seen at NeurIPS 2023
Current status of AI research in drug discovery
It takes an astronomical cost of 1-2 trillion won and more than 10 years of research to create a single new drug. It is a typical high risk high return industry with a very low success rate, but when it succeeds, trillions of won in profits are guaranteed every year.
Recently, the expectation that AI prediction can dramatically reduce the success rate and duration of each stage of drug development has been attracting attention
By utilizing the latest AI technologies based on data accumulated over the past decades, various problems in the drug discovery process, such as drug design, property prediction, and large-scale virtual exploration, can be solved.
Deep learning can be applied not only to small molecule compound-based drug discovery, but also to multi-modalities such as peptide or antibody discovery and protein structure prediction. You can see how deep learning-based AI can be utilized in the drug discovery process in our previous post "Start learning AI drug discovery with these 3 articles".
NerulPS 2023 State of AI in Drug Discovery Research
At NeurIPS 2023, in addition to large language models (LLM), another mega-trend in chemical and biological research, including drug discovery, was highlighted.
On the morning of December 10, the first day of the conference, AstraZeneca (AZ) kicked off the first presentation on drug discovery research with "Artificial Intelligence & Machine learning across the Entire drug development pipeline". The presentation was an introduction to how AI is utilized throughout the entire drug development process.
This was followed by oral and poster presentations on drug development from various organizations, including big tech companies such as Google and MS, global pharmaceutical companies such as AstraZeneca, Genentech, AbbVie, and Merck, and leading universities such as MIT and Harvard.
NeurlPS 2023 ㅣ Drug Discovery Papers and Workshops
Of the total 3584 papers published at NeurIPS 2023, the number of papers according to keyword search was 124 in Drug,** 156 in Protein, 72 in Biology, 32 in Molecule, and 31 in Antibody.
The last two days of the week-long conference are dedicated to subtopic workshops, and this year, a total of five workshops were directly or indirectly related to drug discovery, as shown below. In the first workshop, a graduate student from KAIST (https://wooyoun.kaist.ac.kr/) presented a poster on deep learning-based pharmacophore (PharmacoNet: https://arxiv.org/abs/2310.00681v3).
- New Frontiers of AI for Drug Discovery and Development (https://neurips.cc/virtual/2023/workshop/66504)- Machine Learning in Structural Biology Workshop (https://neurips.cc/virtual/2023/workshop/66513)
- Generative AI and Biology (https://neurips.cc/virtual/2023/workshop/66505)
- Deep Generative Models for Health (https://neurips.cc/virtual/2023/workshop/66495)
- AI for Science: from Theory to Practice (https://neurips.cc/virtual/2023/workshop/66548)
NeurlPS 2023 l Exhibit booths from companies involved in drug discovery
One of the highlights of the conference is the exhibition area of big tech companies. As the world's largest AI conference, NeurIPS is sponsored by many big tech companies, and they operate exhibition booths during the conference. Unlike typical conference booths that market their products, the main purpose of the booth is to recruit AI researchers, so the main developers of each company run the booth and discuss their research with visitors.
Google DeepMind was by far the center of attention at the show.
GNoMe authors, whose work on high-performance new materials has been published in Nature, as well as GEMINI, the recently unveiled AI for weather prediction, gave sessions. Lesser-known Isomorphic Labs researchers also made an appearance at the booth.
(Figure 2). Isomorphic Labs is a subsidiary of Google founded in 2021 for the purpose of drug discovery and spun off from DeepMind. It currently employs about 100 researchers and is said to be focusing on various drug discovery AI research along with the latest version of AlphaFold. Unlike the world's expectations, there has been no detailed introduction of the company's achievements so far, but since 2024, the company's achievements have been announced in the news one by one. As a researcher in the same industry, I have high expectations.
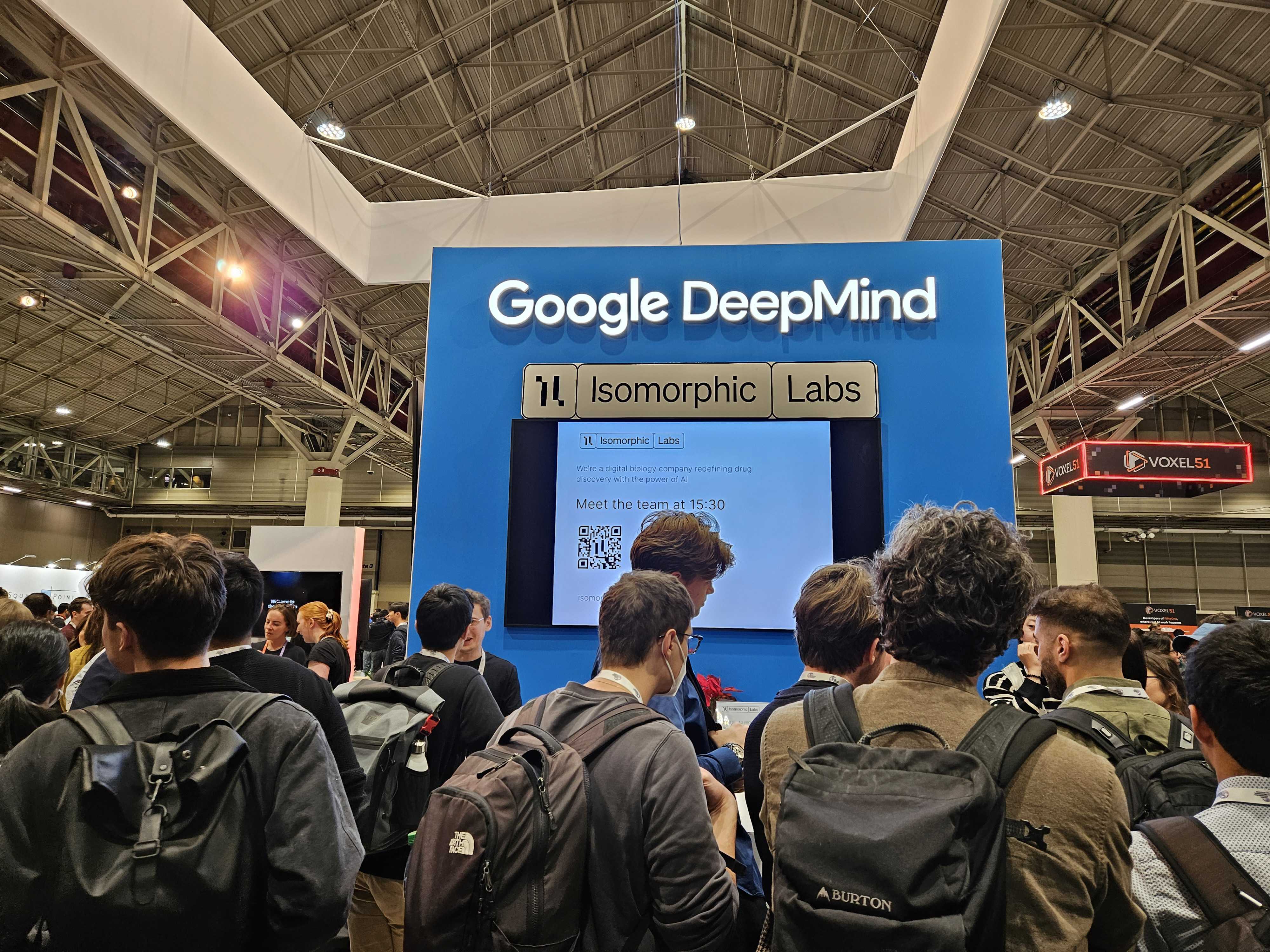
In addition, Valence Labs, the AI research subsidiary of Recursion company, which became famous due to Nvidia's investment, and the Exaone development team of LG AI researchers operated booths to introduce research related to drug development.
NeurIPS 2023 Latest Research Trends in AI Drug Development
Latest Research Trends in AI Drug Discovery, Generative AI (LLM & Diffusion Model)
So, what are the latest research trends in AI drug discovery presented at NeurIPS 2023? If I had to pick one keyword, it would be "Generative AI". Large Language Models, or LLMs, such as GPT and GEMINI, are typical examples of generative AI.
Various studies such as protein design and molecular design have been introduced using LLM. The second most popular generative AI is the Diffusion Model (454 papers published based on the search term diffusion). First introduced by a physicist in 2015, the Diffusion Model has been growing rapidly since 2020 and was the largest growing AI model in 2023.** It was published in Nature in July.
The protein design AI RFdifussion, published in Nature in July ( De novo design of protein structure and function with RFdiffusion, nature, 2023.07.11) is also based on the Diffusion Model. Diffusion Models have pushed out GANs, which once reigned supreme in image generation, and have shown the best performance in image generation, and are now being utilized in various drug discovery studies such as molecular design (Figure 3), protein design (Figure 4), and protein-drug binding structure prediction (Figure 5). Other generative AIs such as GFlowNet have also been utilized.
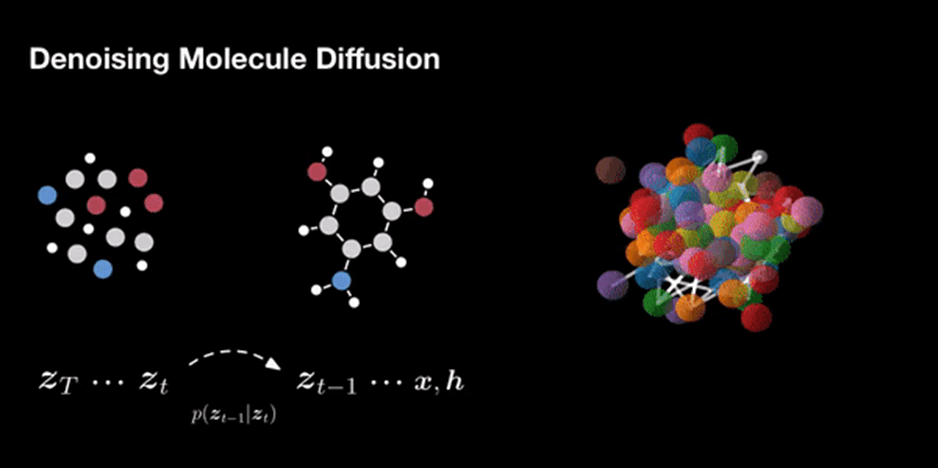
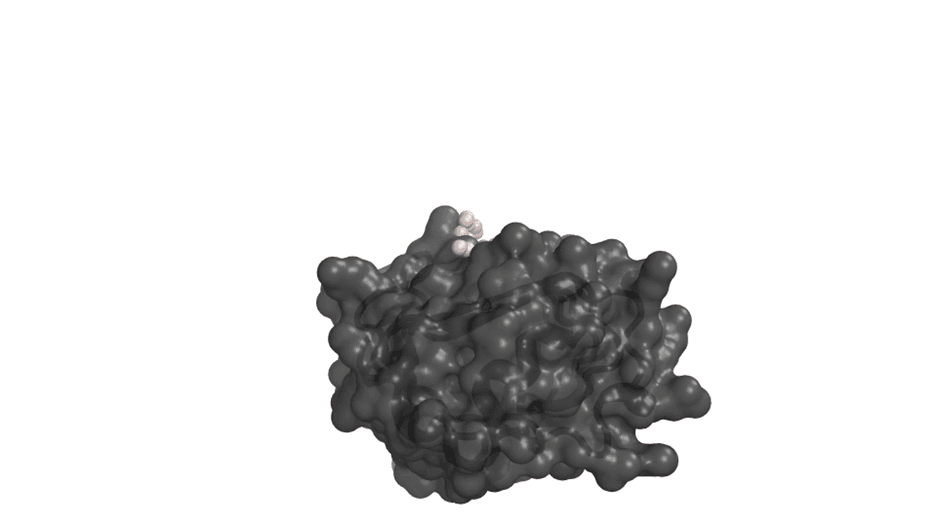
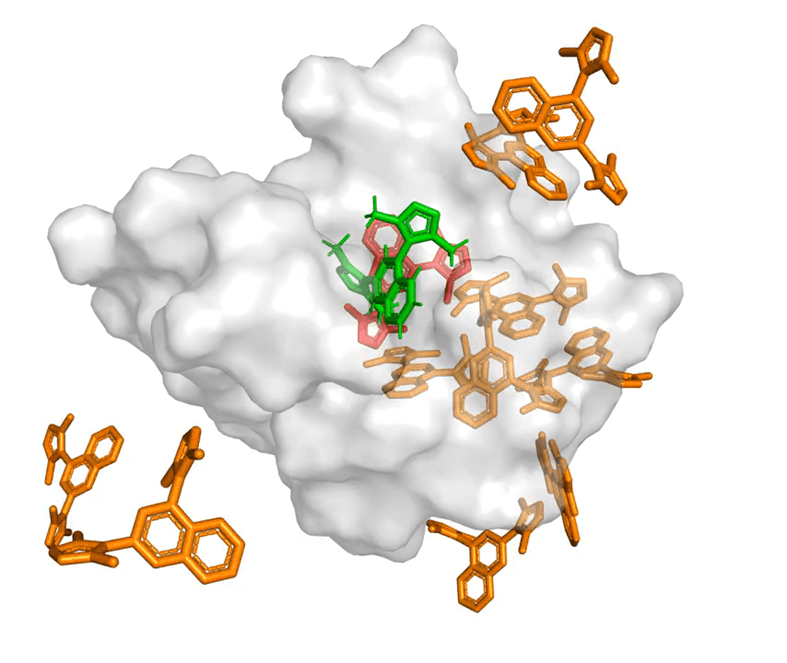
How generative AI will change the future of drug discovery
The rapid growth of generative AI is driving the sophistication of AI techniques in drug discovery.
Even before the application of deep learning, physics-based computational science was actively applied to a variety of problems, including 1) protein structure prediction, 2) protein-drug binding structure and binding force prediction, 3) protein design, and 4) protein-protein interaction prediction.
After decades of research, progress has been slow, but in the past five years, advances in generative AI have led to significant achievements that have exceeded expectations. The first example is AlphaFold2, introduced in 2020, which solved a 50-year-old problem of protein structure prediction with near-perfect accuracy. This conference demonstrated the rapid progress of generative AI as it is applied to various problems that AlphaFold2 could not solve.
On the other hand, it is still rare to see these advances in in silico technology applied to real-world experimental research. On the other hand, it's still very difficult to accurately predict outcomes in the real world, as data scarcity and noise hinder AI performance.
What is clear, however, is that the scientific progress that AI has made in a very short period of time - five years - has been enough to demonstrate its limitless potential. Given the current pace of progress, it's hard to imagine that the next five years won't bring even greater advances, especially since many of the problems that are currently intractable will have been solved in five years, and we'll be tackling them anew.
For example, at this conference, there was a study on AI simulation of the complex protein signaling network in a cell to simulate the whole cell. If such research advances, I am cautiously hopeful that we will move beyond cells to AI simulation of human tissues, organs, and even human systems. I hope that the latest advances in AI technology will be available to all researchers working on drug discovery through the Hyperlab (https://hyperlab.ai/en/).