Hyper Lab Interview with Georgia Institute of technology
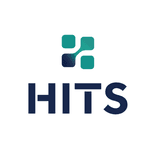
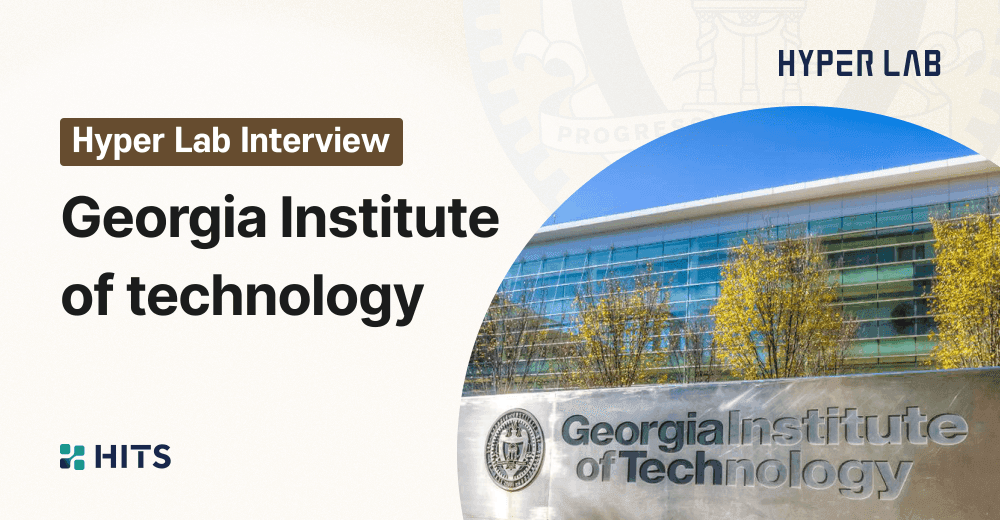
Briefly introduce yourself
My name is Ryan Kern.I'm a fifth-year graduate student at Georgia Tech, and I work in the Oyelere lab. A lot of what we do is medicinal, so drug design, drug synthesis for prostate cancer, and just working on developing new candidates for drugs. We do both experiments and computational drug design.
Do you have any resources you refer to for research?
There's an AutoDock Vina Wiki, which has just basic information about how to get started with the docking and that sort of method. And then for chemical synthesis, there's Reaxys, but that's really for (the case) if you have an idea of a synthesis you want to do, it can show you similar things that people have previously done.
What's the most challenging thing about research? - “The complexity of the correlations between the calculated findings.”
Well, I guess setting synthesis aside because it's just annoying sometimes, but in terms of the computational methods, I would say there's always this level of uncertainty in terms of doing like molecular docking, where you're sort of uncertain how well it will correlate with what you see in an in vitro study. So when it comes to going through and designing all your different compounds and getting a score, you're not sure how well that score will correlate with what you'll see with your cell lines with your enzymatic assays. That’s big question mark sort of guess unanswered as well you know, i would say for most of i docking i do now like autodock vina,...one of my biggest complaints is there is so many different programs import the file you do something and you then export it. import to the another software so it’s managing all the differents.. all your different pdb, different protein, design ligand… much more stressful.
Did Hyper Lab help you solve the above problem? - “Just enter the PDB ID”
Yeah, so Hyper Lab definitely made everything a lot more streamlined, a lot simpler. I think all you needed to do was type in the PDB code, it would get it for you, and then you can draw in your ligand. Hyper Lab is more streamline just by entering the PDB ID. a lot more streamlined in comparison to other docking methods. You just type in the PDB, and then it will prepare it, and from there you just have to import your ligands or draw them, and then it can automatically go through and do the docking, do the prediction of the different physical characteristics.
You mentioned that you correlated the experimental values with the docking values, but what was the actual correlation?
I looked at different classes with different structural components, and within a class, they seemed to correlate with an R^2 of maybe 0.6 to 0.7. But when you started adding different classes together, the correlation wasn't necessarily there. However, for optimizing a drug candidate, you're going to stay within the class, so it should be well-suited for optimizing different drug leads.
Which Hyper Lab feature do you find most useful? - “It would be useful for medicinal chemists or drug discovery researchers specializing in docking.”
I mostly worked with the binding tool, but having the ADME/T prediction right there as well and having everything in a spreadsheet format where it has your structure, your predicted score, and the predicted properties helps keep everything organized. I think it's a very clean way to use it, as opposed to having to go through, make your own spreadsheets, and keep all the information that way. I also liked it when you're doing docking you can also input standards, known inhibitors with known inhibition, and it will generate the graph where you compare the dock score with the actual data values. I think that’s a really cool feature because, with the people who do synthetic medicinal chemistry, there is this worry that you can’t trust molecular docking. So having said that, you can say “Well, here you have this correlation. This should generally correlate with what we expect to see in the lab.”
What were some of the challenges of using Hyper Lab?
I would say there was nothing with the software that frustrated me. again, I was trying to figure out the system with the protein and my ligand, and so I was sort of trying to force it, where I used maybe 10 different PDB's for the same enzyme to see if there was any difference in those and any difference in the docking. But overall, with the software, there's nothing that made me go, "This isn't a good use of my time." It made everything a lot simpler for what I'm trying to do.
Are there any features you were looking forward to when using Hyper Lab, or any areas you felt could be improved?
I would say other than with the result, residue interaction. move around, see how.
i want a little bit more control where you can move around and look at specific protein interactions, like sidechain interactions or residue interactions, in 3D space. Even though you can do it in 2D, it just makes more sense for me to look at it all in 3D. That's one of the features I might want to be added in the future in Hyper Lab.
You mentioned that you correlated the experimental values with the docking values, but what was the actual correlation?
I looked at different classes with different structural components, and within a class, they seemed to correlate with an R^2 of maybe 0.6 to 0.7. But when you started adding different classes together, the correlation wasn't necessarily there. However, for optimizing a drug candidate, you're going to stay within the class, so it should be well-suited for optimizing different drug leads.
How would you recommend Hyper Lab to others interested in small molecule drug discovery?
I would recommend it if they ask for something that's “simple and easy to use”, especially if they don't have the computational know-how or the willingness to learn how to code on their own.