Hyper Lab Interview with Georgia Institute of technology
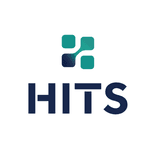
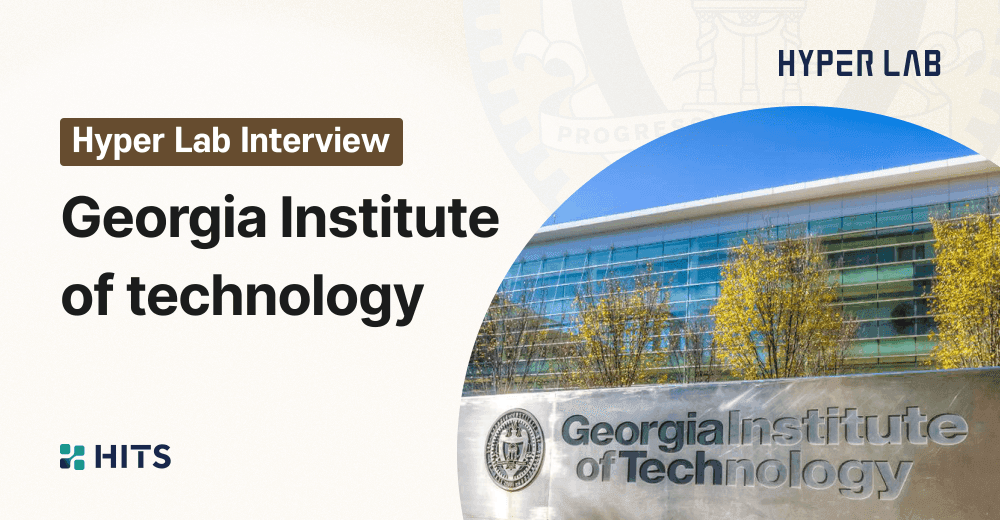
간단한 자기소개 부탁드립니다.
My name is Ryan Kern.I'm a fifth-year graduate student at Georgia Tech, and I work in the Oyelere lab. A lot of what we do is medicinal, so drug design, drug synthesis for prostate cancer, and just working on developing new candidates for drugs. We do both experiments and computational drug design.
제 이름은 Ryan Kern입니다. 조지아 공과 대학교에서 5년째 학업을 이어오고 있으며, Oyelere lab에서 일합니다. 주로 의약화학과 관련된 일을 합니다. 전립성 암을 위한 약물 디자인이나, 약물 합성을 다루고 있으며, 새로운 약물 후보물질을 개발하는 일을 하고 있습니다. 컴퓨터 활용 신약개발도 하지만 직접 실험도 합니다.
연구할 때 참고 하는 자료같은게 있나요?
There's an AutoDock Vina Wiki, which has just basic information about how to get started with the docking and that sort of method. And then for chemical synthesis, there's Reaxys, but that's really for (the case) if you have an idea of a synthesis you want to do, it can show you similar things that people have previously done.
분자 docking 을 위한 자료로는 AutoDockVina wiki가 있습니다. docking 방법에 대한 기본 정보를 제공하죠. 화합물을 합성할때는 Reaxys가 유용합니다. 만약 당신이 합성하고 싶은 아이디어가 있다면, Reaxys를 통해 다른 사람들이 이전에 진행한 유사 합성 결과물을 참고할 수 있죠.
연구에 있어서 가장 어려운 점이 있다면? - ”계산된 연구 결과 간의 상관관계가 복잡해”
Well, I guess setting synthesis aside because it's just annoying sometimes, but in terms of the computational methods, I would say there's always this level of uncertainty in terms of doing like molecular docking, where you're sort of uncertain how well it will correlate with what you see in an in vitro study. So when it comes to going through and designing all your different compounds and getting a score, you're not sure how well that score will correlate with what you'll see with your cell lines with your enzymatic assays. That’s big question mark sort of guess unanswered as well you know, i would say for most of i docking i do now like autodock vina,...one of my biggest complaints is there is so many different programs import the file you do something and you then export it. import to the another software so it’s managing all the differents.. all your different pdb, different protein, design ligand… much more stressful.
가장 어려운 점은 컴퓨터 기반 신약개발 방법의 불확실성을 관리하는 것입니다. 예를 들어 분자 docking에서 계산된 점수와 in vitro 연구 결과 간의 상관관계가 복잡한 경우가 있습니다. 즉 화합물을 디자인하고 점수를 매길 수 있지만 이 점수가 실제 생물학적 활성과 얼마나 일치 할지는 확실치 않죠. 또한 docking과 관련된 다양한 소프트웨어 도구와 파일 형식을 관리하는 것도 스트레스가 큽니다.
하이퍼랩을 위 문제를 해소하는데 도움이 되었나요? - “PDB ID만 입력만 하면 끝”
Yeah, so Hyper Lab definitely made everything a lot more streamlined, a lot simpler. I think all you needed to do was type in the PDB code, it would get it for you, and then you can draw in your ligand. Hyper Lab is more streamline just by entering the PDB ID. a lot more streamlined in comparison to other docking methods. You just type in the PDB, and then it will prepare it, and from there you just have to import your ligands or draw them, and then it can automatically go through and do the docking, do the prediction of the different physical characteristics.
네, 하이퍼랩은 연구 효율성을 크게 향상시켰습니다. 신약개발 과정을 여러모로 단순화해줍니다. PDB ID만 입력하면 필요한 데이터를 가져오고 리간드를 그릴 수 있게 해줍니다. 여러 프로그램과 파일 형식을 관리하는 게 훨씬 간편하고, docking 뿐만 아니라 물성값 예측을 더 직관적으로 잘해낼 수 있습니다.
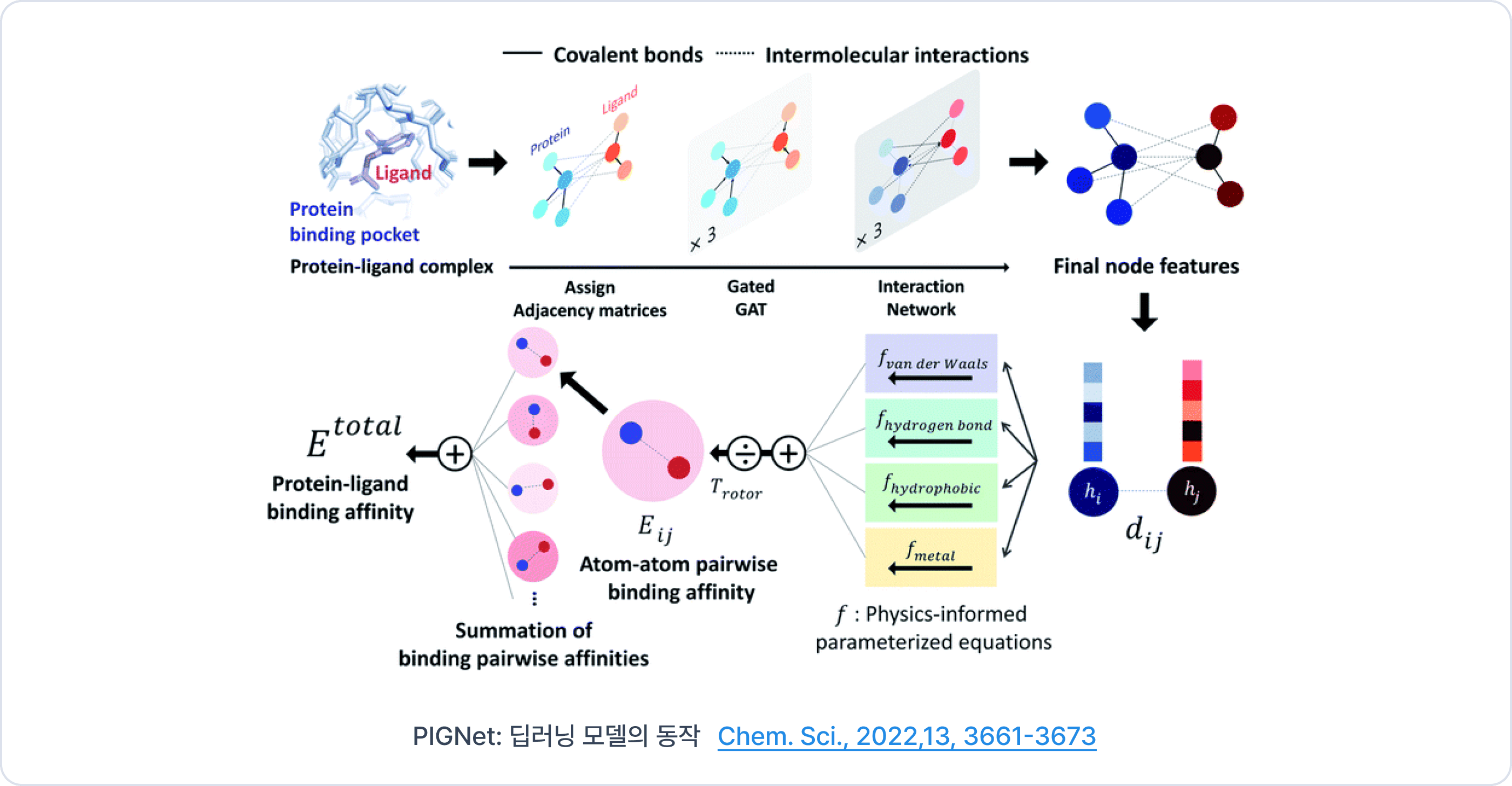
컴퓨터 기반 신약개발 툴에 익숙하다고 말씀주셨는데, 동료분들 중에 혹시 하이퍼랩을 잘 이용할 것 같다고 생각되는 사람이 있나요? - “간편하게 사용할 수 있는 소프트웨어를 찾는 분들”
Yeah, well, I'm the only person in my lab who does the vast majority of the docking. So at Georgia Tech, we have a couple of computational groups who are much more into the weeds of–they want to be able to control everything but aren't really into application of just being able to use a product. I feel like for the people in my lab, who are mostly just interested in getting the thing in and then going into the lab and synthesizing it, it’s much easier to ask, "Hey, will you use the software that's just one click, just easy, straightforward? There's no moving through different software.” Asking them to use something like Hyper Lab would be a lot easier to do than asking them to install Vina, run this Linux script, and do all this stuff.
네, 저는 현재 저희 연구실에서 대부분의 docking 을 맡고 있어요. 조지아 공과대학에는 CADD 팀이 몇개 있는데, 이 팀들은 모든 것을 세밀하게 제어하려고 합니다. 하지만 연구실에서 단순히 특정 제품을 사용하는 것에는 관심이 없죠. 연구실 내 다른 사람들은 주로 실험에 집중하며, 간편하게 사용할 수 있는 소프트웨어 제품을 선호합니다. 이런 사람들에게 Hyper Lab과 같은 소프트웨어는 “한번의 클릭으로 간편하게" 사용할 수 있기 때문에 훨씬 더 쉬운 선택지가 될 수 있습니다. vina 설치하고, linux script 실행하고, 여러 작업을 하는 것보다 하이퍼랩을 이용하는게 훨씬 간편하죠.
하이퍼랩 기능 중 가장 유용하다고 생각되는 건? - “의약화학자나 docking 전문 신약개발 연구원들에게 유용할 것”
I mostly worked with the binding tool, but having the ADME/T prediction right there as well and having everything in a spreadsheet format where it has your structure, your predicted score, and the predicted properties helps keep everything organized. I think it's a very clean way to use it, as opposed to having to go through, make your own spreadsheets, and keep all the information that way. I also liked it when you're doing docking you can also input standards, known inhibitors with known inhibition, and it will generate the graph where you compare the dock score with the actual data values. I think that’s a really cool feature because, with the people who do synthetic medicinal chemistry, there is this worry that you can’t trust molecular docking. So having said that, you can say “Well, here you have this correlation. This should generally correlate with what we expect to see in the lab.”
저는 주로 하이퍼랩에서 바인딩 기능을 사용했지만, ADME/T 예측 값이 함께 제공되는 점이 좋았습니다. 모든 데이터가 스프레드 시트 형식으로 정리되어 있는 점도 매우 유용했습니다. 구조 예측 점수, 물성 예측 값이 잘 정리돼있어서 사용하기 간편했죠. 또한 docking할때 표준 Inhibitor를 입력하면 실제 데이터 값과 docking 점수를 비교할 수 있는 그래프를 생성해주는게 마음에 들었습니다. 하이퍼랩은 합성 하는 의약화학자나 docking하는 신약개발 연구원들에게 매우 유용할 것이라고 생각합니다. 일반적으로는 우리가 기대하는 결과에 잘 부합하는 것 같습니다.
하이퍼랩을 사용하면서 불편했던 점은 있었나요?
I would say there was nothing with the software that frustrated me. again, I was trying to figure out the system with the protein and my ligand, and so I was sort of trying to force it, where I used maybe 10 different PDB's for the same enzyme to see if there was any difference in those and any difference in the docking. But overall, with the software, there's nothing that made me go, "This isn't a good use of my time." It made everything a lot simpler for what I'm trying to do.
소프트웨어 자체에서 불편했던 점은 없었습니다. 단지, 저는 단백질과 리간드의 결합 정도를 알아보려고 10가지 다른 PDB를 넣어서 사용해보기도 했어요. 같은 enzyme 내에서 docking 결과에 어떤 차이를 가져오는지 실험하려했습니다. 이러한 과정은 전반적으로 제가 하는 docking 작업을 훨씬 간편하게 만들어주었고 시간 낭비라는 생각은 들지 않았습니다.
하이퍼랩을 사용할때 기대했던 기능이나, 개선이 더 필요하다 느낀 부분이 있나요?
I would say other than with the result, residue interaction. move around, see how.
i want a little bit more control where you can move around and look at specific protein interactions, like sidechain interactions or residue interactions, in 3D space. Even though you can do it in 2D, it just makes more sense for me to look at it all in 3D. That's one of the features I might want to be added in the future in Hyper Lab.
단백질 상호작용, 예를 들어 side chain 상호작용이나 잔기 상호작용을 3D 공간에서 살펴볼수있는 좀 더 세부적인 제어 기능이 있으면 좋겠습니다. 2D에서도 가능하지만 3D로 보는 게 더 직관적이라고 생각해 이 기능이 하이퍼랩에 추가되면 좋겠어요.
실험 값과 docking 값과의 상관 관계를 확인했다고 하셨는데 실제로 상관관계는 어땠나요?
I looked at different classes with different structural components, and within a class, they seemed to correlate with an R^2 of maybe 0.6 to 0.7. But when you started adding different classes together, the correlation wasn't necessarily there. However, for optimizing a drug candidate, you're going to stay within the class, so it should be well-suited for optimizing different drug leads.
다양한 구조를 가진 클래스들을 살펴본 결과, 같은 클래스 내에서는 R square 값이 약 0.6에서 0.7 정도로 상관관계가 있는 것으로 판단되었습니다. 하지만 서로 다른 클래스를 함께 추가하면 상관관계가 뚜렷하지 않았습니다. 그러나 보통 약물 후보를 최적화할때는 같은 클래스 내에서 진행하므로 하이퍼랩은 hit-to-lead에 최적화된 도구라고 생각합니다.
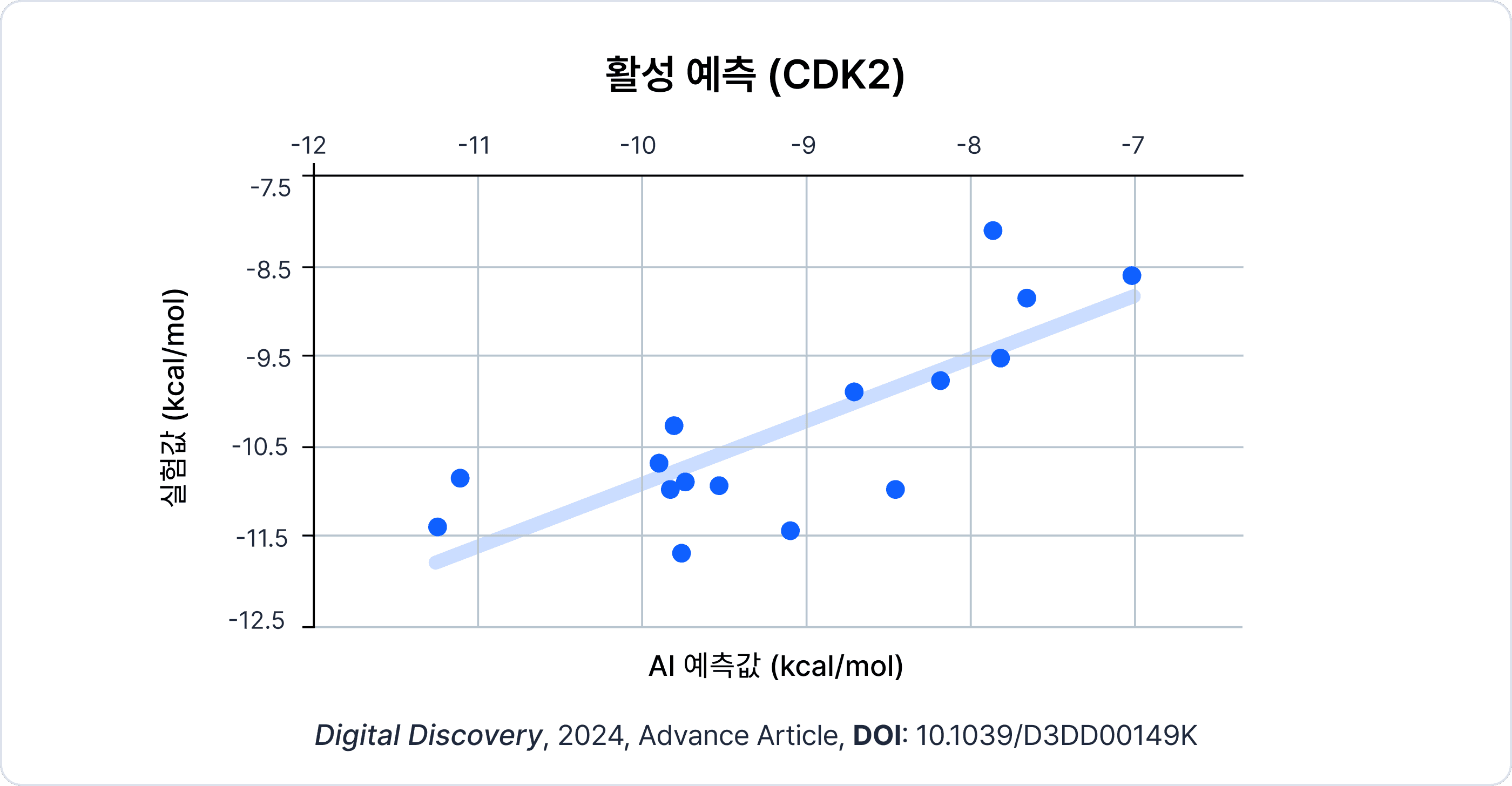
저분자 화합물 신약개발에 관심 있는 다른사람들에게 하이퍼랩을 추천해준다면?
I would recommend it if they ask for something that's “simple and easy to use”, especially if they don't have the computational know-how or the willingness to learn how to code on their own.
간단하고 사용하기 쉬운 도구를 찾는다면 하이퍼랩을 추천합니다. 특히 컴퓨터 기반 지식이나 코딩을 배우고 싶지 않은 분들에게 유용하다고 자신합니다.